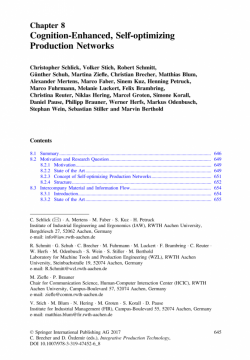
Abstract
8.1 Summary
Companies are facing a wide range of challenges in the field of customer demand, dynamic environment conditions, and quality problems regarding the management level. Therefore, they need to adapt themselves and their processes to the dynamic environment conditions. Examples are movements in customer demand, resched- ules in supply, as well as turbulences in networks. Thus, high process efficiency and a wide availability of information are characteristics of a successful production management.
Hereby, the management of negative consequences is challenging. Negative consequences can lead to wrong decisions in the planning processes caused by poor communication or conventional solution approaches based on centralized planning methods. This leads to companies that react slower and more inflexible on internal and external disturbances and consequently to an increasing gap between reality and intention.
Another result of volatile markets and a demand for high flexibility is a fast-changing production environment that requires frequent adjustments of pro- duction control configuration to ensure companies produce at competitive costs. However, companies rarely check and adjust their production control configuration due to the high effort for analyzing the current situation and calculating or simulating alternatives.
On the shop floor, the complexity of automated and semiautomated production systems results in a variety of different objectives that have to be considered in the discrete manufacturing industry. In regard to the planning and control of production processes, the underlying optimization problem is multidimensional. In order to reduce efforts, especially for product orientation and mass customization, methods to automatically adjust to new environments and new objectives are required.
Dealing efficiently with the dynamic environment of production is one of the most challenging tasks of production planning and control (PPC) in high-wage countries. The cluster of excellence “Integrative Production Technologies for High Wage Countries” at RWTH Aachen University addresses these opportunities and challenges and aims at resolving the “polylemma of production” which spans along two dilemmas: plan-oriented versus value-oriented production and scale versus scope. To compete in globalized markets, enterprises must solve this polylemma and growing interconnectivity of technical systems between different tiers of a production network and across companies is a possible solution. While enterprise resource planning (ERP) systems and manufacturing execution systems (MES) are increasingly penetrating manufacturing companies and automated processes increase companies’ productivity by optimizing the flow of resources and information, there are—and always will be—human operators in the loop that maintain control, take responsibility, and who must have a profound understanding of aspects not modeled in these systems.
The main result, which has been achieved in previous phases of the project, is a cybernetic model structuring a self-optimizing production management on its different levels, capable of coping with dynamics and to continuously adjust itself. Based on these results, the project addresses now the comprehensive application of self-optimizing planning and control on all levels of the value chain. This implies the integration of self-optimizing control loops on cell level with those addressing the PPC as well as supply chain and quality management aspects and will lead to an improvement of planning decisions on different levels of the production network. A specific focus is on the consideration of human decisions during the production process when elaborating the cognitive model. To establish socio-technical control loops, it is necessary to understand how human decisions in diffuse working processes as well as cognitive and affective abilities form the human factor within production processes are made.
To enable companies to react fast to these challenges, means of self-optimization have been developed for the different levels of the value chain (supply chain, production control and shop floor).
One main result is a reference model for the intercompany material and information flow between companies. In order to enable the system and subsystem to optimize dynamically and independently, logic from the control theory were adopted and transferred to enable a real-time coordinated PPC between companies on the level of the supply chain. Principles from the viable system model (VSM) have been transferred to the developed concept which serves as a basis for self-optimizing control loops. In order to study the interrelationships between human diversity, novel VSM-based decision support systems, and the technical parts of production systems and the industrial Internet, a novel research framework through business simulation games is proposed. The business simulations used originate from the VSM modeling and allow the identification and quantification of human factors in socio-technical production environments, serving as benchmarks to evaluate decision support systems and their user interfaces. In this research, it is demonstrated that usability of enterprise software has a quantifiable positive influence on effectiveness and efficiency in decision scenarios. It was further established that some users are able to compensate for the negative effect of bad user interfaces, but that the costs of poor usability grow quickly for others if the amount and complexity of information increase.
On the level of the production control, the simulation tool WoPS 4.0 has been developed in order to support the necessary analyses and helping companies with optimally adjusting their production control configuration. This tool consists mainly of architecture for the automatic generation of simulation models and can be connected in a self-optimizing control loop to PPC systems of manufacturing companies for permanently checking production control performance. The production supervisor is only provided with information from the system if action is needed. This results in a highly reduced effort for production supervisors and the possibility to adjust production control configuration in a superior fashion. By enabling companies to produce economically, an important contribution to strengthen companies in high-wage countries is made.
Regarding the shop-floor level, research was carried out to develop self-optimization production lines with incorporate human decision behavior. Thus, a cognitive automation for robotic assembly processes was developed. By means of generic assembly knowledge and cognitive decision-making, the system is not only able to adjust itself to environmental changes, but also to make comprehensible decisions that conform to the operator’s expectations. Further research has shown that introducing anthropomorphic movements for assembly robots can additionally decrease the mental stress of the operator and increase the predictability of the system behavior. Thereby, the human is able to trust the system, which is advantageous especially in case of direct human–robot cooperation.
Furthermore, the developed Viable MES (VMES) focuses on the modeling and integration of a production control unit for automated production systems that are structured according to the VSM. The system is able to incorporate different goals in different hierarchies of the overall factory. Its viability enables a further inte- gration of socio-technical aspects regarding the planning and coordination for the overall factory as well as the specific processes. One major challenge in processes with high cycle times is to increase the overall equipment effectiveness (OEE)— mainly by focusing the technical availability. A common approach is to apply condition monitoring together with predictive maintenance in order to avoid unplanned downtimes. Nevertheless, process interruptions due to wear do not lead to component failure in the first place. The cloud-based integration and therefore combination of a broad data basis, powerful algorithms, and machine, respectively, component models promise significant improvements for wear detection and pro- cess adjustment.
Companies are facing a wide range of challenges in the field of customer demand, dynamic environment conditions, and quality problems regarding the management level. Therefore, they need to adapt themselves and their processes to the dynamic environment conditions. Examples are movements in customer demand, resched- ules in supply, as well as turbulences in networks. Thus, high process efficiency and a wide availability of information are characteristics of a successful production management.
Hereby, the management of negative consequences is challenging. Negative consequences can lead to wrong decisions in the planning processes caused by poor communication or conventional solution approaches based on centralized planning methods. This leads to companies that react slower and more inflexible on internal and external disturbances and consequently to an increasing gap between reality and intention.
Another result of volatile markets and a demand for high flexibility is a fast-changing production environment that requires frequent adjustments of pro- duction control configuration to ensure companies produce at competitive costs. However, companies rarely check and adjust their production control configuration due to the high effort for analyzing the current situation and calculating or simulating alternatives.
On the shop floor, the complexity of automated and semiautomated production systems results in a variety of different objectives that have to be considered in the discrete manufacturing industry. In regard to the planning and control of production processes, the underlying optimization problem is multidimensional. In order to reduce efforts, especially for product orientation and mass customization, methods to automatically adjust to new environments and new objectives are required.
Dealing efficiently with the dynamic environment of production is one of the most challenging tasks of production planning and control (PPC) in high-wage countries. The cluster of excellence “Integrative Production Technologies for High Wage Countries” at RWTH Aachen University addresses these opportunities and challenges and aims at resolving the “polylemma of production” which spans along two dilemmas: plan-oriented versus value-oriented production and scale versus scope. To compete in globalized markets, enterprises must solve this polylemma and growing interconnectivity of technical systems between different tiers of a production network and across companies is a possible solution. While enterprise resource planning (ERP) systems and manufacturing execution systems (MES) are increasingly penetrating manufacturing companies and automated processes increase companies’ productivity by optimizing the flow of resources and information, there are—and always will be—human operators in the loop that maintain control, take responsibility, and who must have a profound understanding of aspects not modeled in these systems.
The main result, which has been achieved in previous phases of the project, is a cybernetic model structuring a self-optimizing production management on its different levels, capable of coping with dynamics and to continuously adjust itself. Based on these results, the project addresses now the comprehensive application of self-optimizing planning and control on all levels of the value chain. This implies the integration of self-optimizing control loops on cell level with those addressing the PPC as well as supply chain and quality management aspects and will lead to an improvement of planning decisions on different levels of the production network. A specific focus is on the consideration of human decisions during the production process when elaborating the cognitive model. To establish socio-technical control loops, it is necessary to understand how human decisions in diffuse working processes as well as cognitive and affective abilities form the human factor within production processes are made.
To enable companies to react fast to these challenges, means of self-optimization have been developed for the different levels of the value chain (supply chain, production control and shop floor).
One main result is a reference model for the intercompany material and information flow between companies. In order to enable the system and subsystem to optimize dynamically and independently, logic from the control theory were adopted and transferred to enable a real-time coordinated PPC between companies on the level of the supply chain. Principles from the viable system model (VSM) have been transferred to the developed concept which serves as a basis for self-optimizing control loops. In order to study the interrelationships between human diversity, novel VSM-based decision support systems, and the technical parts of production systems and the industrial Internet, a novel research framework through business simulation games is proposed. The business simulations used originate from the VSM modeling and allow the identification and quantification of human factors in socio-technical production environments, serving as benchmarks to evaluate decision support systems and their user interfaces. In this research, it is demonstrated that usability of enterprise software has a quantifiable positive influence on effectiveness and efficiency in decision scenarios. It was further established that some users are able to compensate for the negative effect of bad user interfaces, but that the costs of poor usability grow quickly for others if the amount and complexity of information increase.
On the level of the production control, the simulation tool WoPS 4.0 has been developed in order to support the necessary analyses and helping companies with optimally adjusting their production control configuration. This tool consists mainly of architecture for the automatic generation of simulation models and can be connected in a self-optimizing control loop to PPC systems of manufacturing companies for permanently checking production control performance. The production supervisor is only provided with information from the system if action is needed. This results in a highly reduced effort for production supervisors and the possibility to adjust production control configuration in a superior fashion. By enabling companies to produce economically, an important contribution to strengthen companies in high-wage countries is made.
Regarding the shop-floor level, research was carried out to develop self-optimization production lines with incorporate human decision behavior. Thus, a cognitive automation for robotic assembly processes was developed. By means of generic assembly knowledge and cognitive decision-making, the system is not only able to adjust itself to environmental changes, but also to make comprehensible decisions that conform to the operator’s expectations. Further research has shown that introducing anthropomorphic movements for assembly robots can additionally decrease the mental stress of the operator and increase the predictability of the system behavior. Thereby, the human is able to trust the system, which is advantageous especially in case of direct human–robot cooperation.
Furthermore, the developed Viable MES (VMES) focuses on the modeling and integration of a production control unit for automated production systems that are structured according to the VSM. The system is able to incorporate different goals in different hierarchies of the overall factory. Its viability enables a further inte- gration of socio-technical aspects regarding the planning and coordination for the overall factory as well as the specific processes. One major challenge in processes with high cycle times is to increase the overall equipment effectiveness (OEE)— mainly by focusing the technical availability. A common approach is to apply condition monitoring together with predictive maintenance in order to avoid unplanned downtimes. Nevertheless, process interruptions due to wear do not lead to component failure in the first place. The cloud-based integration and therefore combination of a broad data basis, powerful algorithms, and machine, respectively, component models promise significant improvements for wear detection and pro- cess adjustment.
Schlick, C., Stich, V., Schmitt, R., Schuh, G., Ziefle, M., Brecher, C., Blum, M., Mertens, A., Faber, M., Kuz, S., Petruck, H., Fuhrmann, M., Luckert, M., Brambring, F., Reuter, C., Hering, N., Groten, M., Korall, S., Pause, D., Brauner, P., Herfs, W., Odenbusch, M., Wein, S., Stiller, S., Berthold, M.: Cognition-Enhanced, Self-optimizing Production Networks. In: Brecher, C. and Özdemir, D. (eds.) Integrative Production Technology - Theory and Applications. pp. 645–743. Springer International Publishing, Berlin, Heidelberg (2017).
Related Project(s):